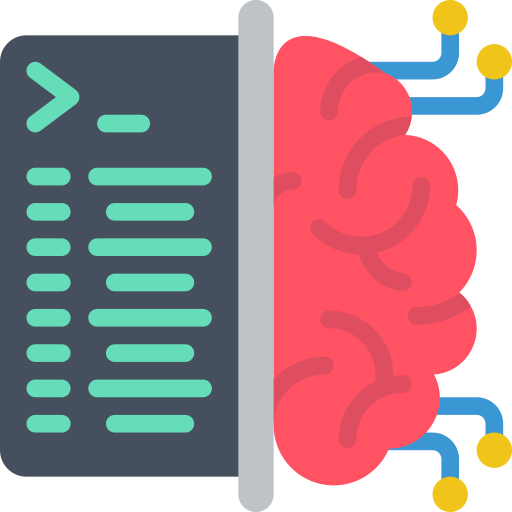
Modeling & Machine Learning Interview
20 of 63 Completed
Introduction
Overview
How Much Machine Learning?
Types of Machine Learning Interview Questions
Introduction
Machine learning and modeling concepts consist of some of the most basic fundamentals in data science. Given that it’s a rapidly evolving field, machine learning is almost always in need of updates. Therefore, as a data scientist, it’s important to keep up with the latest trends and technologies that are constantly being released.
Modeling interview questions and the machine learning interview are many times an abstraction for testing a candidate’s experience in the field, as well as determining to what degree a data scientist or machine learning engineer can critically apply theory towards a business goal.
As we go through each framework, interview question, and machine learning concept, it’s worth remembering that machine learning and modeling interview questions are ultimately indicative of two things:
- A candidate’s past experience working with machine learning.
- The capacity to memorize concepts and apply them towards solutions the interviewer is looking for.
It’s best to use this section as a litmus test for your own knowledge in machine learning and understanding, but note that this section is not meant for academics or Phd graduates trying to learn entirely new concepts.
Instead we’re going into dive into some core interviewing concepts around the machine learning interview including:
The six parts of a machine learning case study interview
Frameworks for tackling any sort of machine learning question
The differences between different types of machine learning interview questions
System design and productionizing machine learning models
31%
CompletedYou have 43 sections remaining on this learning path.