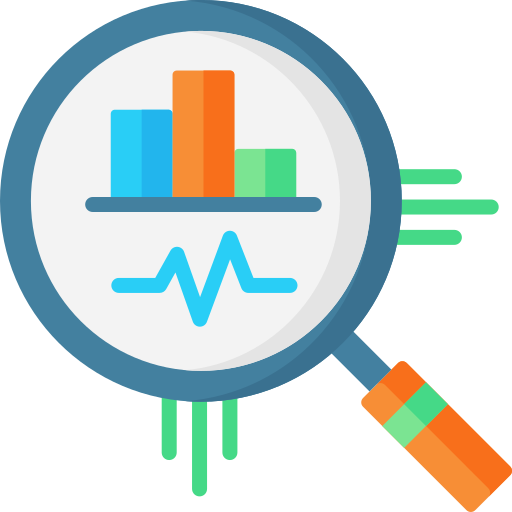
Data Science Interview
92 of 257 Completed
Introduction
Fundamentals of Hypothesis Testing
The Z and t test
Z and t Tests
Two-sample Tests
Proportion Testing
Different Parcel Effectiveness
Analysis of Variance (ANOVA)
Hundreds of Hypotheses
Non-parametric Tests
Non-Normal AB Testing
Introduction
The Problem
When you read the news, you might hear about a sample statistic like “the average person spends more than 2 hours using social media a day”. You might wonder, how do they know that? Clearly, you can’t survey every person on earth that uses social media, and requesting user data from every social media company would be a daunting and error-prone task.
Instead, the reporters likely took a sample of social media users and asked them how long they used social media on a typical day. But sampling can be tricky. Consider the following two samples of the time (in hours) that users use social media:
- (1,1,1,1,7)
- (1,2.5,2.5,2.5,2.5)
Both of these groups on average, spend more than 2 hours using social media on a regular date. But one person in the first sample group actually individually spends more than 2 hours browsing social media on a typical day.
Now obviously (good) organizations would use larger sample sizes, but the point stands that it’s not very clear how you could tell if a conclusion drawn from a sample is “bad”. For example, is the it “wrong” to conclude from the following sample that the average person uses social media for more than two hours a day?
Hard to say
Hypothesis Testing
Hypothesis Testing is a systematic way to make inferences from sample data. It can help solve questions like the one above for data sets, but many more, including
- Is a certain political poll trustworthy?
- Is there a significant difference between medical outcomes of a control and test group?
- Is the average income of citizens higher between two towns?
- Is there greater variation in the time one cohort spends on our website compared to another cohort
And much, much more.
Hypothesis Testing in Interviews and Industry
Since the advent of digital technologies, hypothesis testing has become less prominent than in the base because our data sets have gotten so huge that we automatically assume our samples are “good.” To an extent, this ambivalence is justified: sample sizes of 10000+ give near-certainty that the sample statistic obtained from them are reliable.
However, there are still cases where even large companies like Amazon or Google face small sample sizes:
- A/B tests with a low test group proportion
- New product launches
- Control group research
In these cases, hypothesis testing is still very relevant. Additionally, because hypothesis testing is such an important idea in the development of statistics, you are likely to get asked about the basics of it even if your role will rarely involve performing actual tests.
Course Outline
In the next section, we will go over the fundamentals of hypothesis testing. Then, we will go over a series of the most important tests any good person working in a data-related industry should understand. In order, they will be:
- -tests and -tests, for inferring about sample means
- Two-sample tests, for comparing samples means
- Proportion tests, including the -test, for inferring about and comparing sample proportions (percentages)
- Analysis of variance (ANOVA) tests, for comparing variances between samples
- Ranked tests, for inferring about and comparing sample median
35%
CompletedYou have 165 sections remaining on this learning path.